As the race toward fully autonomous vehicles (AVs) accelerates, the demand for precise, large-scale data annotation becomes more critical than ever. These intelligent machines rely on a vast amount of labeled data to make real-time driving decisions—identifying pedestrians, traffic signs, lane markings, and more. But how do we generate and maintain the quality of that data at the scale required?
The answer lies in a hybrid model: combining automation with human-in-the-loop (HITL) systems to optimize efficiency and accuracy. In this article, we’ll explore how the blend of automation and human insight is shaping the future of data annotation, enabling safer and smarter self-driving systems.
The Backbone of AV Learning: Why Data Annotation Matters
Data is the fuel of artificial intelligence, and in the case of AVs, annotated data is what teaches machines how to drive. Every decision an autonomous vehicle makes—whether it’s stopping at a red light or avoiding a cyclist—is informed by thousands of hours of sensor data, including camera footage, LiDAR scans, radar signals, and GPS logs.
For AVs to interpret this data correctly, it must first be labeled with high precision. This involves tagging objects (like pedestrians, cars, and traffic signs), classifying lane lines, drawing bounding boxes, and identifying environmental conditions such as weather or time of day. The quality of this labeled data directly influences the performance of the vehicle’s perception models.
Automation in Annotation: Speed and Scalability
Automated data labeling tools leverage AI to detect and annotate objects within datasets without—or with minimal—human intervention. These tools are trained on previously labeled examples and can rapidly process vast quantities of data.
Key benefits of automated labeling include:
- Speed: Machines can label thousands of frames in a fraction of the time it takes humans.
- Consistency: Algorithms maintain a uniform approach, reducing variation in labeling.
- Cost-efficiency: Automation significantly reduces the labor required for large-scale projects.
However, automation is not a silver bullet. While AI can handle routine and repetitive labeling tasks, it often struggles with edge cases—rare or unusual scenarios that AVs must still be able to recognize and respond to. Think of a person in a costume crossing the road, or a fallen tree partially obstructing a lane. These rare occurrences are critical to train for, yet challenging for automated systems to detect accurately.
Where Human Insight Meets Machine Speed
To overcome the limitations of pure automation, human-in-the-loop systems introduce expert annotators into the process. Humans validate, correct, or refine the output from automated tools, ensuring the final data is both accurate and contextually appropriate.
This hybrid method is especially critical in the field of autonomous vehicle data labeling, where even the smallest labeling error could translate into a serious safety risk. HITL ensures that datasets used to train AV systems are not only large but also rich in context, accuracy, and completeness.
Humans bring a level of understanding that machines can’t—like interpreting gestures from traffic officers or recognizing subtle environmental cues. Their input helps cover edge cases, improve model training, and continuously refine automated systems.
Scaling Up: Hybrid Annotation Pipelines
To meet the data demands of AV development, companies are increasingly turning to hybrid annotation pipelines—customized workflows that integrate automated tools with human quality assurance.
A typical hybrid pipeline might look like this:
- Initial pass by AI models: Raw sensor data is fed into an automated annotation system trained on existing datasets.
- Human review layer: Annotators inspect and correct outputs, flagging problematic cases or unknown scenarios.
- Feedback loop: The system learns from corrections, gradually improving its accuracy over time.
- Continuous auditing: Regular spot checks and audits ensure ongoing quality and compliance with annotation standards.
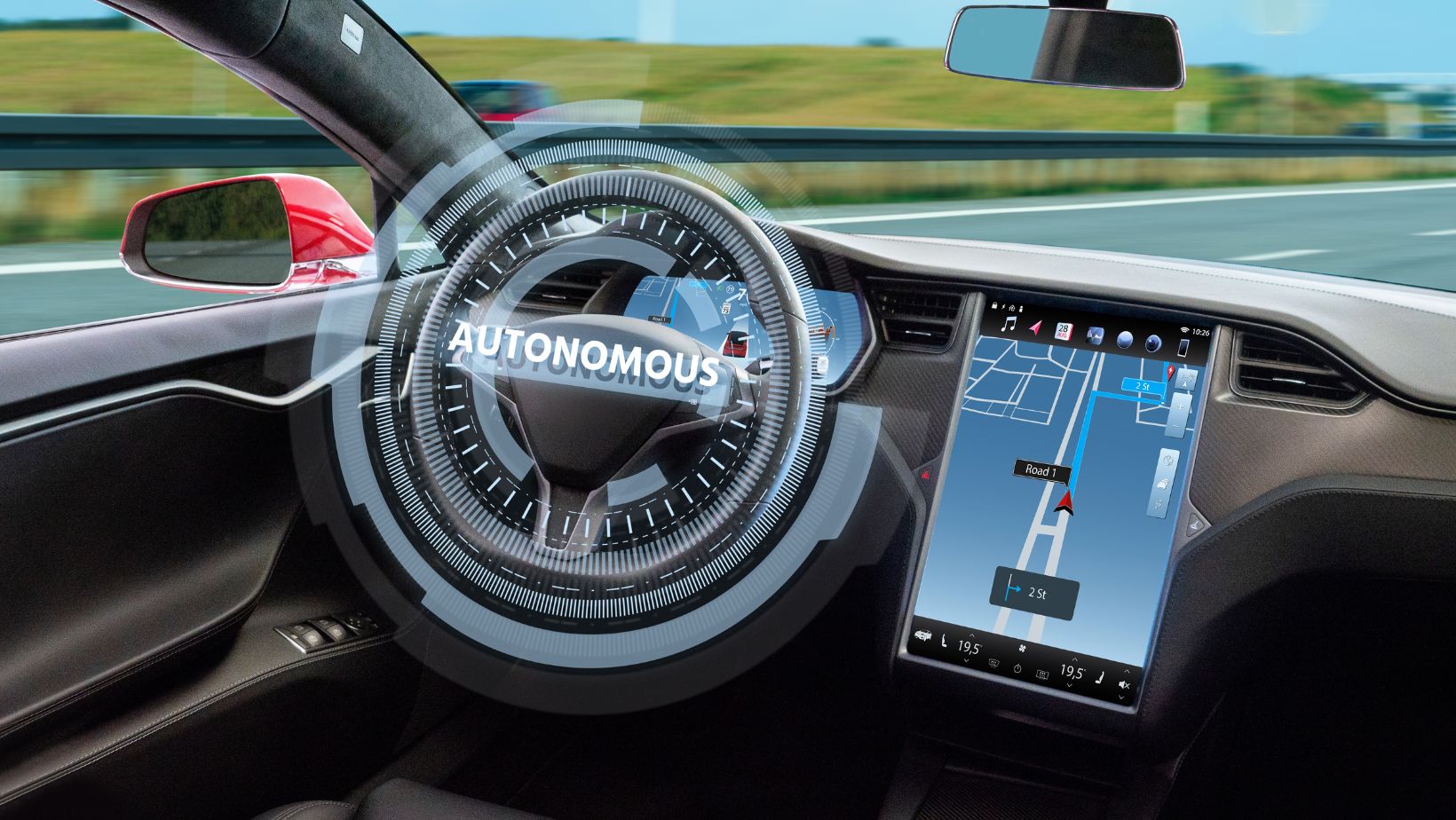
This combination not only ensures high-quality annotations but also significantly reduces the turnaround time for preparing training datasets. As more data flows through the pipeline, the automation component becomes smarter—allowing human effort to be increasingly focused on high-complexity cases.
The Role of Tooling and Infrastructure
Beyond the human and algorithmic elements, the success of large-scale annotation also hinges on the tools and platforms used. Annotation software must support a wide variety of sensor types and annotation methods, from 2D bounding boxes to 3D LiDAR segmentation and temporal labeling across video sequences.
Modern platforms are built with scalability in mind, offering:
- Cloud-based collaboration for distributed teams.
- Real-time dashboards to track labeling progress and quality metrics.
- Version control systems to manage iterations and feedback loops.
- Integration with model training pipelines for seamless dataset updates.
These tools are crucial for managing the complexity and volume of data involved in training AV systems.
Conclusion: Collaboration for the Road Ahead
The journey to fully autonomous driving is long and complex, but data annotation remains one of the most foundational steps in that evolution. Pure automation, while powerful, is not yet capable of replacing the contextual reasoning and nuanced understanding that humans bring to the table. Conversely, relying solely on human labor is unsustainable at scale.
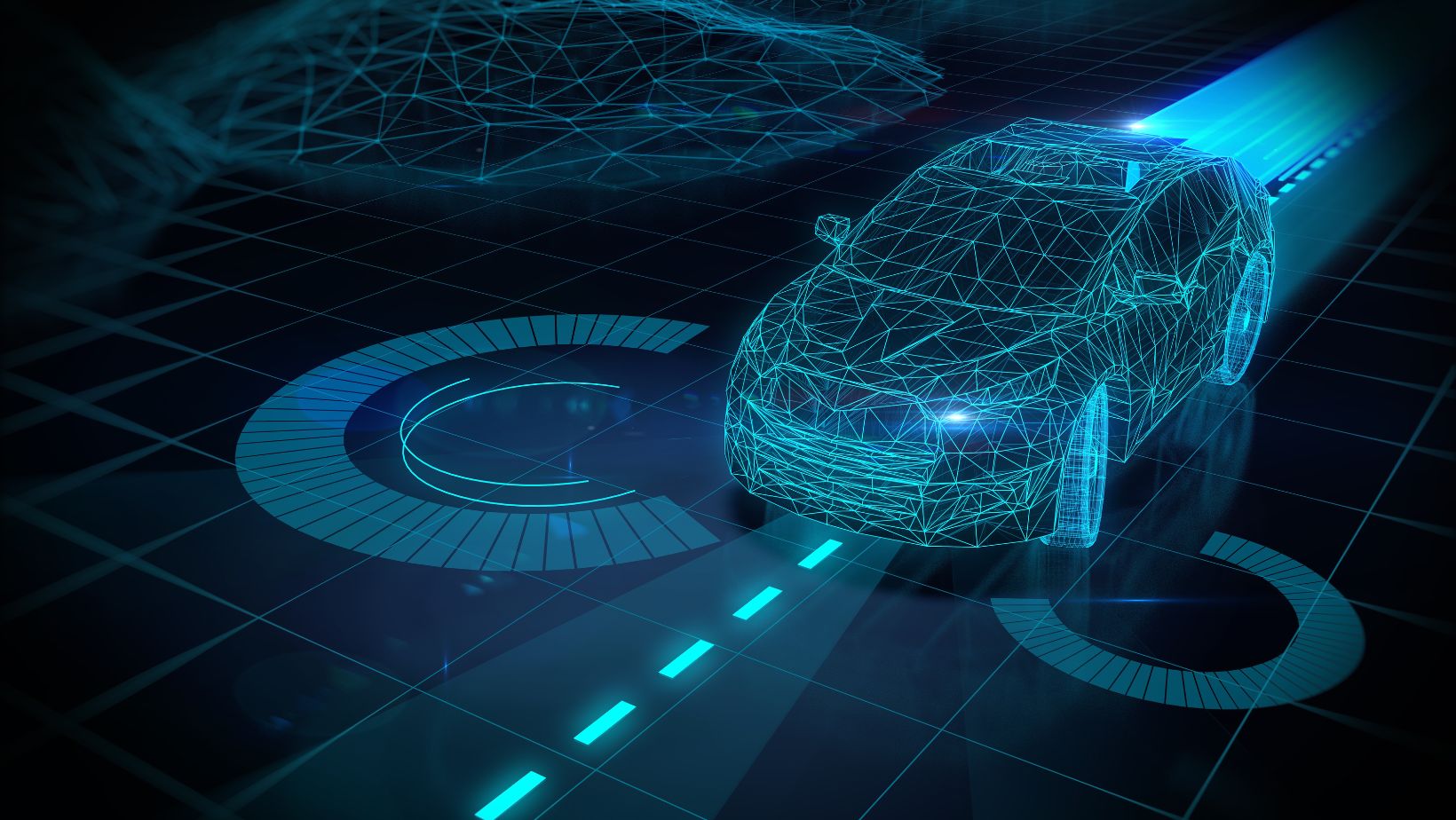
The future lies in hybrid approaches—automated systems that handle the bulk of annotation work, augmented by skilled humans who guide and correct them. This synergy ensures high-quality datasets, faster development cycles, and ultimately, safer and more reliable autonomous vehicles.
In a field where every pixel and point cloud matters, the marriage of automation and human insight is not just beneficial—it’s essential for scaling the future of mobility.