The world of technology is developing at an incomparable pace, wherein its pursuits, the amalgamation of machine learning and edge computing occur. Conventionally, most machine learning models have relied on the mighty cloud servers for processing huge amounts of data; however, recent developments are pushing these models toward the very creation of data to more sovereign edge devices. Edge computing moves computation and data storage closer to the data source, thereby unlocking new possibilities for real-world machine learning deployment with reduced latency and increased privacy.
In this article, we shall see the opportunities opened up by combining machine learning with edge computing and some of the unique challenges that are thrown up by such a combination. As this field evolves, the benefits and hurdles of ML on the edge can help a business make a proper determination on how those sets of technologies should be best integrated into operations.
Opportunities in Machine Learning at the Edge
ML at the edge will revolutionize many industries, from faster and more efficient to highly secure applications. Following are some of the key opportunities brought about by this convergence:
Real-Time Decision Making
One of the most significant advantages of deploying machine learning models on edge devices is the possibility to make real-time decisions locally. Applications that require decisions in milliseconds, such as autonomous cars, industrial automation, or healthcare monitoring systems, would be intolerant to delays while transferring data to cloud servers for processing and analysis. With the use of edge computing, processing is done locally, enabling instant analysis and action.
For example, in a self-driving car, the onboard systems must make split-second decisions to detect obstacles, pedestrians, or other vehicles. Machine learning models deployed on edge devices enable this rapid response without the latency that comes with sending data back and forth to a remote server. This real-time capability is crucial for safety and efficiency in such applications.
Reduced Latency and Bandwidth Usage
This approach minimizes the amount of data that needs to be transferred to some central servers, since processing is already happening at the device level. The result is reduced latency, which is quite critical to applications that demand quick responses. Minimizing data transfers to the cloud also reduces bandwidth consumption, thus reducing operations’ overall costs while improving network resource utilization.
Edge computing paired with machine learning is particularly useful in remote areas with limited or unreliable connectivity. By enabling local data processing, edge devices can still function effectively even when cloud connectivity is compromised. This is why industries like agriculture, where connectivity is often sparse, are beginning to leverage machine learning at the edge to analyze soil quality, weather conditions, or crop health without depending on continuous cloud access.
In doing so, the ability to leverage these capabilities for organizations translates to the need to consider the help of a machine learning development services provider. Such a provider has the requisite know-how in developing and optimizing the ML models for deployment on edge devices, focusing on achieving the required latency, power, and performance constraints.
Improved Privacy and Security
Another critical benefit that machine learning at the edge has to offer involves improved privacy. Considering the prevailing period where data privacy emerges as a key issue, processing the information locally on an edge device implies that sensitive information needn’t traverse over the internet to a centralized server. This may drastically reduce the chances of data breaches or unauthorized access, particularly in applications that involve healthcare, finance, and smart home devices.
Consider wearable health devices using machine learning to track health metrics of an individual. The analysis can be done right on the device. This means private health information of a user is not sent to a remote server. In this case, the risk of exposure for private health information is minimized. Because edge computing keeps data processing local, it provides an additional layer of security, thus making it an attractive option for industries that are conscious about privacy.
Challenges of Machine Learning at the Edge
While combining machine learning with edge computing bears a lot of advantages, there are also many significant challenges that must be resolved in order for it to become a feasible solution for a wide range of applications. Now, let us look at some of these challenges in detail:
Limited Computing Resources
This is one of the most interesting challenges that stand in the way of deploying machine learning at the edge: the computational power of edge devices seriously lags behind that of cloud servers. It is usual for IoT sensors, smartphones, and embedded systems to have lower CPU, memory, and/or even power limits.
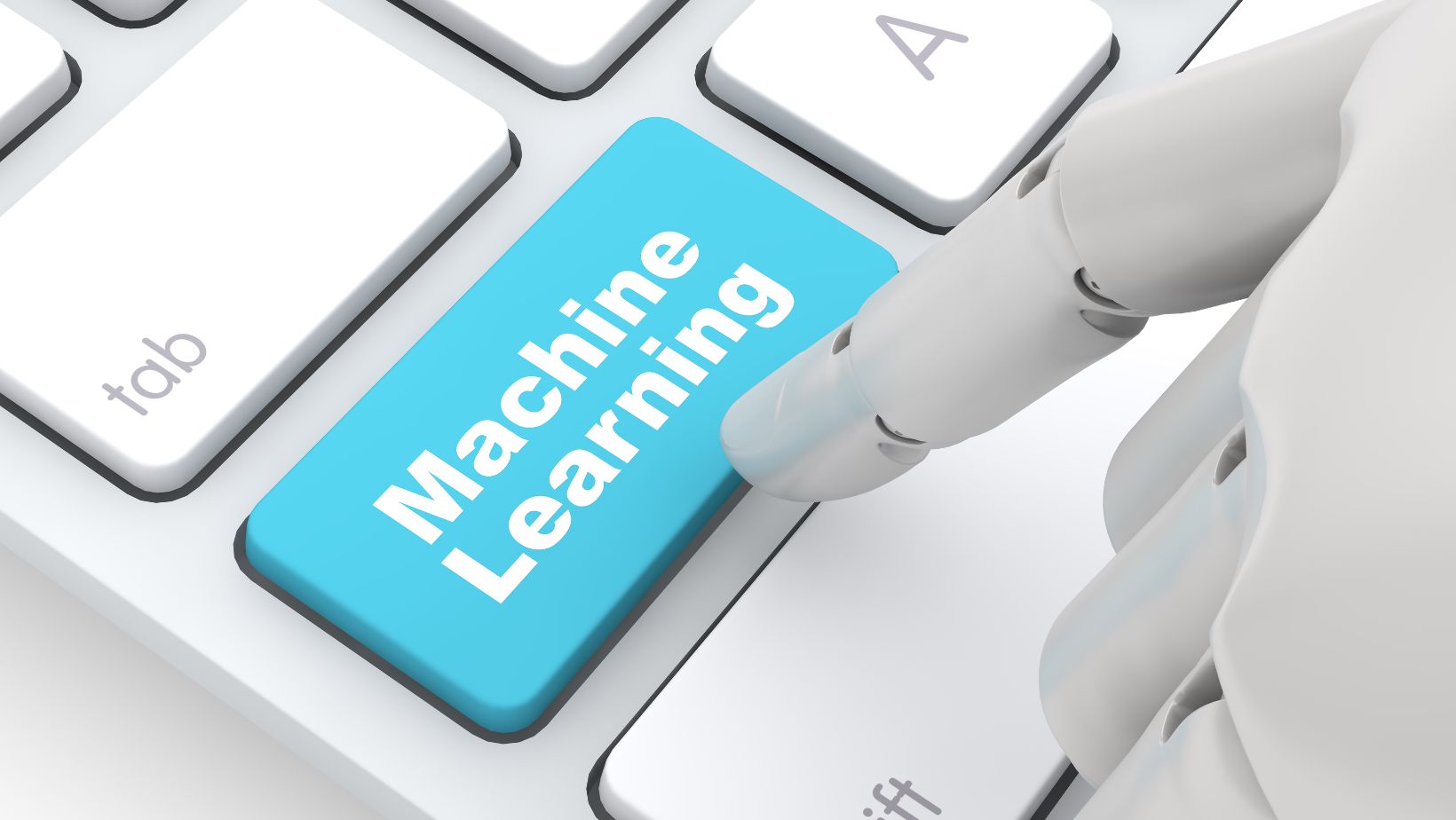
Thus, sophisticated deep learning models need a lot of such resources, and hardly any such models can be run on such devices.
To surmount this hurdle, developers should focus on model optimization techniques such as pruning, quantization, and knowledge distillation. Pruning involves eliminating redundant parameters within a model, hence its size and computational requirements are reduced. Quantization involves reducing the precision of the model parameters, which may contribute to reducing memory usage and processing demands. Other approaches, such as knowledge distillation, where a smaller, lightweight model learns from a much larger, more complex one but retains much performance while being deployable on edge devices, have been explored.
Energy Efficiency
Most edge devices, especially the ones utilized in IoT applications, are powered by batteries, which entails strict energy consumption. Running machine learning models on these types of devices quickly drains their batteries, thus making energy efficiency a major concern. Consequently, it is expected for developers to design models and algorithms that are optimized for the lowest power consumption.
To that effect, there’s an increasing interest in using hardware accelerators, such as dedicated AI chips, to solve this problem. Such chips are made to carry out ML computations more power-efficiently than general-purpose processors and therefore consume much less energy. In any case, adding these specialized hardware components to edge devices often raises costs and further complicates the development process.
Model Deployment and Management
Deploying machine learning models to edge devices is more complicated compared to cloud deployment. First of all, edge devices are often scattered across different locations and may be difficult to manage and keep up to date with models. The scenario could be such that thousands of devices, each with different hardware configurations and different connectivity, have to be upgraded with the new model version by an organization.
These deployments must be effectively managed by mechanisms such as model versioning, monitoring, and performing OTA updates. The use of containerization tools such as Docker can ensure consistency in the model environment across devices. This guarantees that such an ML-driven edge-based computing system will be consistent with reliable performance over time.
Machine Learning in Edge Computing: The Future
The next advances in machine learning and edge computing will ease this process further for the deployment of powerful ML models at the edge. With more efficient algorithms, along with new developments in hardware, including AI accelerators and neuromorphic chips, the way is open for more complex and capable models to be deployed at the edge.
Of note is also an increasing utilization of federated learning. Federated learning involves a number of edge devices collaborating to train a machine learning model without the need for sharing raw data. This approach addresses not only privacy concerns but also greatly diminishes the amount of data over-transmission, hence further enhancing the efficiency of edge-based ML systems. This is of concern for industries like healthcare, which require high levels of data privacy and where the gathering of data is usually highly distributed.
Conclusion
The combination of machine learning and edge computing offers the exciting prospect of taking powerful, real-time, and secure AI closer to the origin of the data creation. With instant decision-making, lessened latency, and boosted privacy, machine learning from the edge will radically change industries from healthcare and automotive to agriculture and smart cities. However, significant challenges that need to be overcome before full utilization of edge-based ML include those in the areas of limited computational resources, energy efficiency, and model deployment complexities.
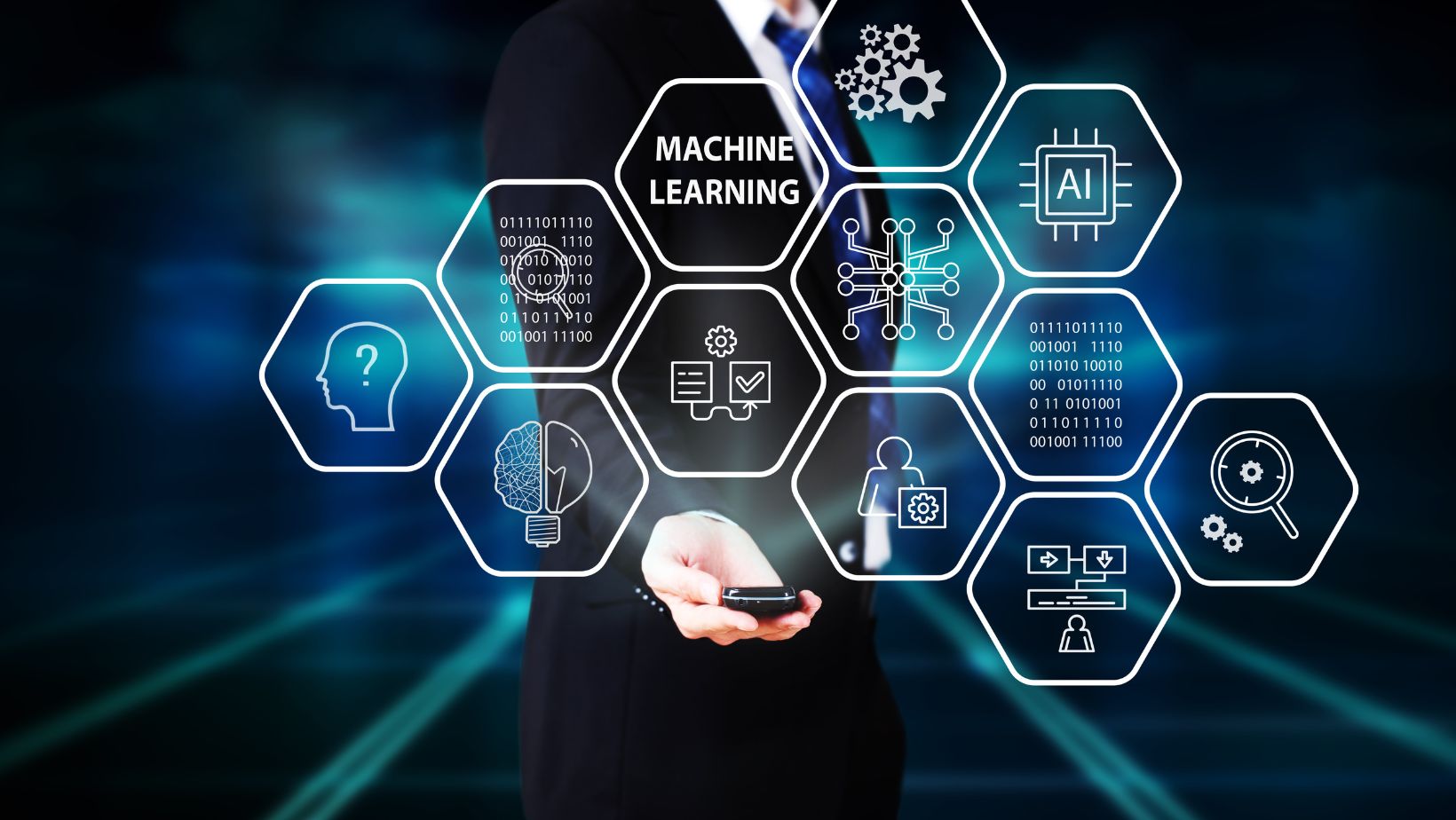
Organizations that want to harness machine learning at the edge will have to contemplate partnering with experts in machine learning development services in order to master these challenges effectively. Understanding these opportunities and overcoming these obstacles are two ways through which businesses will be able to tap into the power of machine learning on the edge for solutions that are not only effective but also quicker and more secure.