In the rapidly evolving landscape of artificial intelligence, Retrieval Augmented Generation (RAG) is emerging as a groundbreaking technology. RAG seamlessly integrates the capabilities of AI with real-time information retrieval, thus enhancing enterprise data analysis. By bridging traditional AI capabilities with dynamic, real-time data insights, RAG stands poised to transform how businesses interact with data.To comprehend the impact of RAG, one must first understand what is retrieval augmented generation and how it serves as an excellent resource for a detailed overview of this technology.
Core Technological Foundations
At its essence, RAG is built upon three key technological pillars:
- Machine Learning Integration: RAG leverages advanced machine learning algorithms to improve data retrieval processes, ensuring that the most relevant information is accessed quickly and efficiently. For a deeper dive into these technologies, consider exploring a comprehensive machine learning overview.
- Natural Language Processing Mechanisms: By utilizing sophisticated NLP techniques, RAG can interpret and generate human-like text responses, making it invaluable for applications that require an understanding of nuanced queries.
- Dynamic Information Retrieval Techniques: Unlike traditional static systems, RAG dynamically pulls in real-time data from various sources, ensuring that the information provided is current and contextually relevant.
Evolution from Traditional AI Models
Traditional AI models often face limitations due to their static nature and reliance on pre-existing data sets. RAG addresses these challenges by offering:
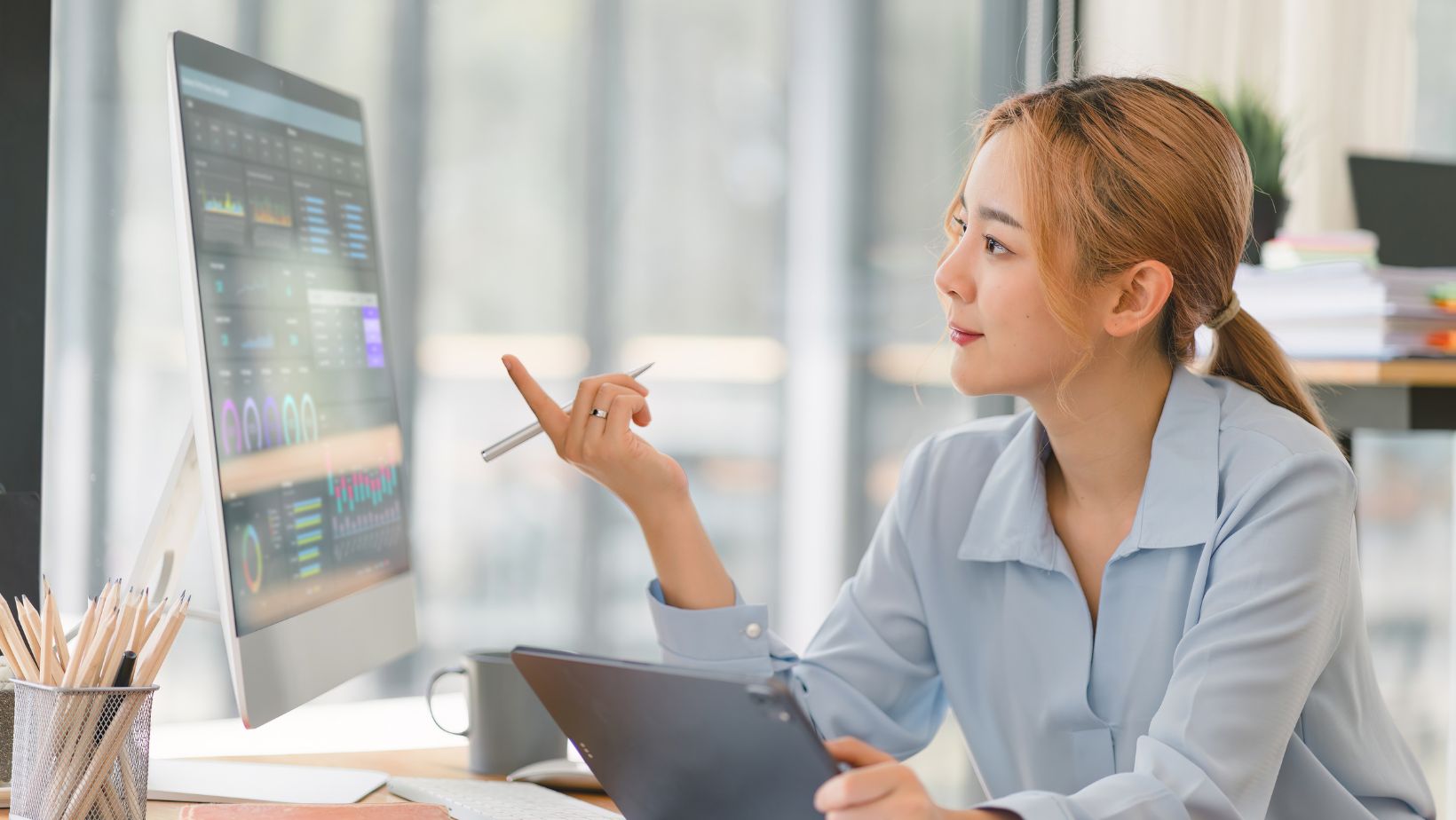
- Adaptive Information Processing: RAG systems can adapt to new data inputs in real-time, providing more accurate and timely insights.
- Real-time Contextual Understanding: By constantly updating its data sources, RAG can offer insights that are not only timely but also contextually accurate, enhancing decision-making processes within enterprises.
Enterprise Applications of RAG
The transformative potential of RAG in enterprise environments is vast, offering significant enhancements in data analysis workflows, decision-making processes, and cross-departmental information integration.
Business Intelligence and Analytics
RAG is revolutionizing business intelligence by enabling:
- Real-time Insights Generation: With RAG, businesses can access real-time data insights, thus making informed decisions faster than ever before.
- Predictive Analytics Enhancement: By integrating RAG with predictive analytics, companies can foresee trends and patterns with greater accuracy.
- Complex Data Interpretation: RAG simplifies the interpretation of complex datasets, allowing businesses to extract actionable insights efficiently. For more on business intelligence strategies, refer to this business intelligence strategy guide.
Customer Experience Optimization
In the realm of customer experience, RAG offers:
- Personalized Interaction Strategies: RAG systems can tailor interactions based on real-time data, providing customers with a personalized experience.
- Dynamic Customer Support: By using RAG, customer support systems can dynamically access the latest information to resolve queries more effectively.
- Contextual Recommendation Systems: RAG can enhance recommendation systems, offering suggestions that are relevant to the customer’s current context and needs. This approach significantly boosts customer satisfaction and loyalty.
Implementation and Future Potential
While the benefits of RAG are clear, implementing this technology involves certain challenges and strategic considerations.
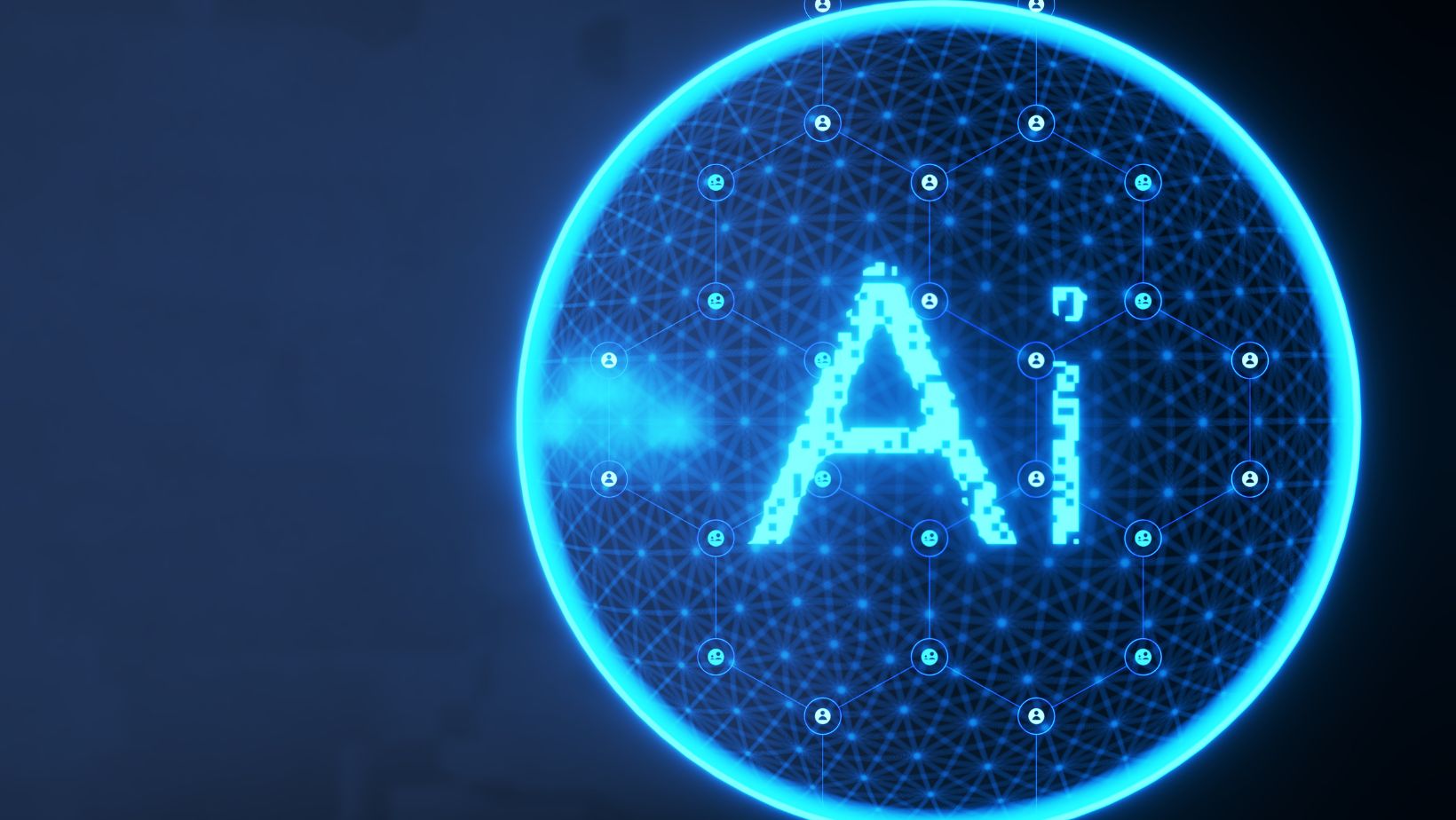
As RAG continues to evolve, it presents exciting future opportunities for enterprises.
Technical Architecture Considerations
To successfully integrate RAG, enterprises must consider:
- Data Source Integration: Ensuring seamless integration with diverse data sources is critical for RAG’s effectiveness.
- Scalability Requirements: As the volume of data grows, RAG systems must be scalable to handle increased demand without compromising performance.
- Security and Privacy Protocols: Given the sensitive nature of enterprise data, robust security and privacy measures are essential to protect against potential threats.
Future Innovation Trajectories
The future of RAG is promising, with several avenues for development:
- AI Model Sophistication: As AI models become more sophisticated, RAG’s capabilities will expand, offering even more advanced data retrieval and analysis functions.
- Cross-Industry Adaptation Potential: Beyond enterprise data analysis, industries such as healthcare, finance, and retail can leverage RAG for various applications.
- Ethical AI Development: As RAG becomes more widespread, ensuring ethical AI development will be crucial for maintaining trust and integrity.
Retrieval Augmented Generation is a transformative force in real-time data analysis, offering enterprises unprecedented capabilities in data processing and decision-making. As RAG technology continues to evolve, its potential to drive innovation across industries remains limitless.