A feature store serves as a crucial component in the machine learning (ML) lifecycle, acting as a centralized repository for storing, managing, and sharing features across various models and projects. As the complexity of ML workflows increases, the need for structured and accessible feature data becomes paramount.
A feature store not only enhances collaboration among data scientists and engineers but also ensures consistency and scalability in feature engineering. By facilitating the reuse of high-quality feature sets, organizations can accelerate their ML initiatives, reduce redundancy, and improve model performance, ultimately leading to more data-driven decision-making and a competitive edge in the market.
Streamlining Feature Engineering Processes
Streamlining the feature engineering process is one of the primary advantages of implementing a feature store. By providing a structured environment for defining, creating, and storing features, data scientists can significantly reduce the time spent on feature extraction. This efficiency is critical as it allows teams to focus more on model development rather than the labor-intensive process of feature generation. Additionally, this streamlined process can minimize errors that often arise when manually producing features for different models.
With a feature store, practitioners are enabled to define a feature’s metadata, including its origin, transformation logic, and usage scenarios. This detailed documentation fosters better understanding and collaboration among team members. With clarity on the components of a feature store, data scientists can quickly identify and reuse features to power new models with ease. Also, this process enables organizations to consistently build models in a standardized and reproducible manner.
Ensuring Feature Consistency Across Models
Consistency is vital in machine learning, as differing datasets can lead to dramatically different model performances. A feature store ensures that features are consistently defined and used across various models and projects. By standardizing features, teams can avoid discrepancies that may arise from manual feature extraction and preprocessing steps. This uniformity is crucial, particularly for organizations that deploy multiple models or conduct A/B testing, as it simplifies comparisons and analysis.
Maintaining a consistent feature set allows for better governance and compliance with data policies. A feature store typically provides version control for features, ensuring that every model can reference the specific version of a feature used in its training. This capability not only improves reproducibility but also promotes trust in the data science process, as stakeholders can verify that everyone is working with the same foundational data.
Promoting Collaboration Between Teams
One of the core benefits of a feature store is its ability to promote collaboration among teams within an organization. Data scientists, data engineers, and product teams can all access a shared repository of features, making it easier to align on project goals and share best practices. This collaboration enables more efficient work processes as data scientists can easily discover and integrate features developed by their peers rather than starting from scratch.
In addition, this centralized approach facilitates interdisciplinary communication. Data scientists can collaborate with domain experts to create features that reflect real-world complexities, while engineers can provide insights on data pipeline considerations. This cross-functional collaboration is instrumental in enriching the feature set and ensuring that it meets both technical and business needs, ultimately optimizing the development pipeline.
Enhancing Scalability of ML Operations
As organizations grow and their data needs evolve, scalability becomes a critical concern for machine learning operations. A feature store directly addresses this need by supporting the dynamic scaling of features without compromising their quality or accessibility. With a robust feature store, teams can efficiently manage an expanding library of features while ensuring that they remain relevant and usable across multiple models.
Scalable feature stores can accommodate a high volume of incoming data and diverse feature types, including categorical, numerical, and time-series data. This capability allows organizations to keep pace with rapid changes in data sources and consumer behavior, ensuring that their models are always enriched with the latest inputs. Consequently, businesses can respond more swiftly to market demands and maintain a competitive edge.
Facilitating Real-time Data Access
The ability to access and respond to data in real-time is crucial. Feature stores make it possible to provide features in real time for models that demand immediate decision-making, such as recommendation systems or fraud detection algorithms. This functionality enhances the performance of machine learning applications, allowing them to utilize the most current data available.
Additionally, feature stores can integrate with streaming data sources, enabling businesses to build real-time applications that react instantly to incoming information. This capability not only maximizes the effectiveness of machine learning models but also helps businesses leverage timely insights, leading to improved customer experiences and more informed decisions.
Supporting Model Monitoring and Governance
A feature store plays a significant role in monitoring and governance by enabling organizations to track feature usage and performance over time. By maintaining detailed logs of features and their impact on model outcomes, teams can quickly identify which features contribute to success and which do not. This ongoing evaluation helps ensure that models remain robust and efficient as business environments change.
Robust governance protocols can be established within a feature store to comply with legal and ethical guidelines regarding data usage. Access controls, auditing features, and automatic logging of feature changes ensure that there is a clear trail for accountability. This layer of governance is paramount as it fosters trust in machine learning initiatives and fulfills regulatory requirements.
Driving Cost Efficiency in ML Projects
Implementing a feature store can lead to significant cost savings in machine learning projects over time.
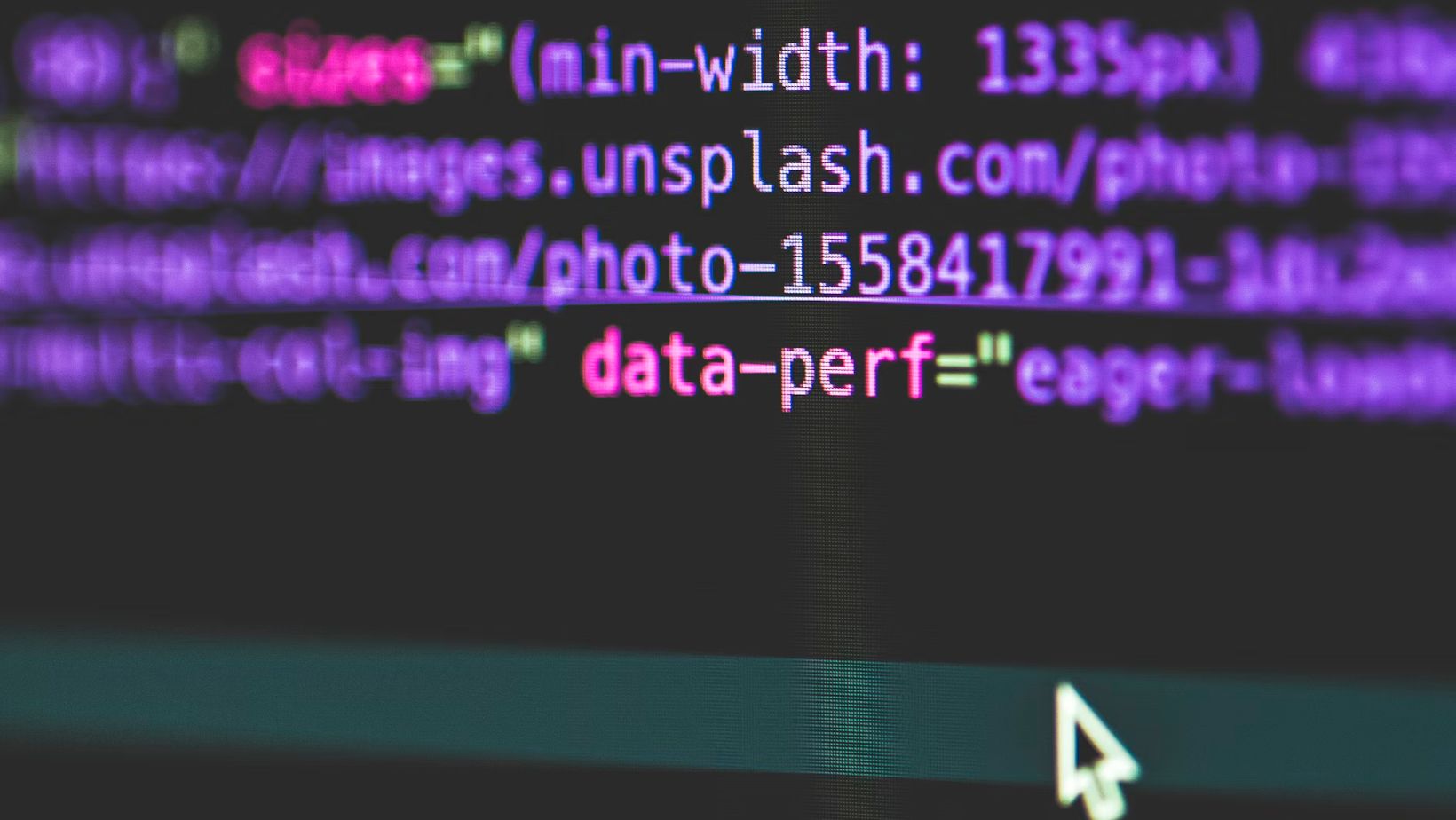
By reducing redundancy in feature development and streamlining workflows, organizations can optimize the use of their engineering resources. Instead of duplicating efforts to generate similar features for different models, teams can leverage existing features, resulting in a more efficient allocation of time and budget.
Additionally, the enhanced model performance resulting from high-quality and consistent features can lead to better business outcomes, further justifying the initial investment in a feature store. As organizations maximize their return on investment, they can utilize saved resources to innovate and explore new opportunities, solidifying their position in the competitive landscape.
A feature store is an invaluable asset for organizations looking to enhance their machine-learning capabilities. By streamlining feature engineering, ensuring consistency, promoting collaboration, and enhancing scalability, feature stores empower teams to develop robust models more efficiently. The ability to access real-time data and maintain governance furthers the operational integrity of machine learning projects, while the cost efficiencies achieved can drive significant business value.
As the field of machine learning continues to evolve, investing in a feature store positions organizations to leverage data effectively, innovate continuously, and maintain a competitive advantage in the marketplace. Embracing this vital component of the ML lifecycle is essential for those aiming to achieve sustainable success in data-driven decision-making.